Predicting Readmission for Heart Failure
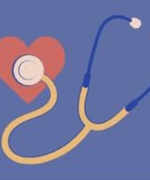
Researchers at the University of California Davis developed an artificial
intelligence algorithm to predict readmission of patients with heart failure,
enabling patients and clinicians can make appropriate treatment decisions
long before hospitalization is required.
Heart failure is a chronic condition where the heart cannot pump enough blood to meet the body’s demands for blood and oxygen. About 1 in 50 people are diagnosed with heart failure today and, with an aging population, this number is expected to increase. Patients admitted to hospital with heart failure have long and frequent hospital stays, with over one fifth of patients needing to be readmitted 30 days after discharge. However, there is currently no straightforward way to accurately identify which patients will require readmission before symptoms progress.
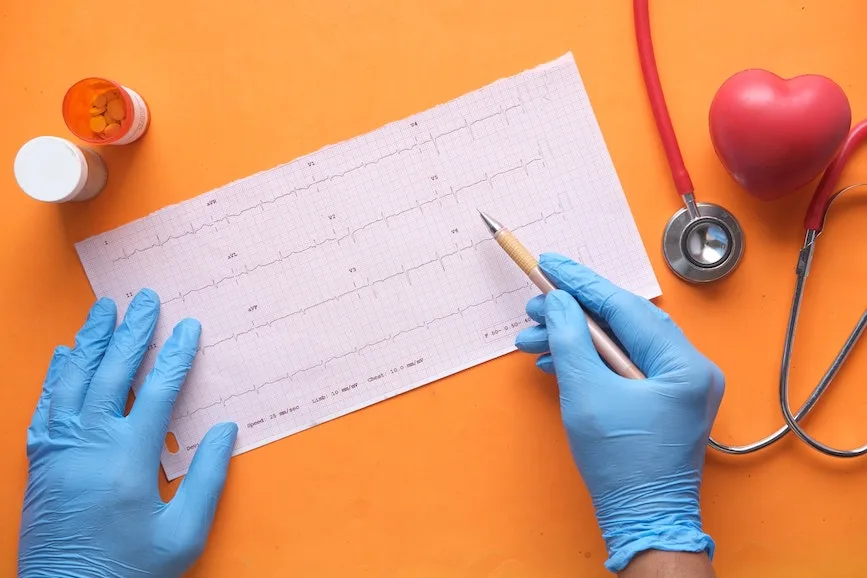
Dr. Katherine Kim at the University of California Davis and a team of research collaborators tackled this challenge of identifying which patients are likely to require readmission soon after discharge. Five years prior to the commencement of the project, over 120 clinicians, patients, and other stakeholders came together to discuss which area(s) of health were of utmost priority. During this time, Dr. Kim and colleagues started talking to health care leaders to see if and how these priorities would impact the healthcare system. They identified that telemonitoring had not been as impactful as expected, and the stakeholders emphasized that heart failure was an urgent priority, and thus the project to create and integrate a technology that could predict readmission following heart failure was born. From the beginning, this project was designed with the needs of the health care community at the forefront.
The team worked with clinicians, patients, health care leadership, and hospital leadership to design the research project. They partnered with Rayid Ghani, a data scientist at the University of Chicago and Data Science for Social Good to develop an artificial intelligence (AI) algorithm which could accurately assess and predict readmission through patient data. This research required the integration of new techniques in artificial intelligence using large and complex data sets with the practical needs of a particular segment of the health care system, with a goal of achieving considerably better health outcomes. After successful completion of Phase I, the team secured additional funding to collaborate with visualization expert Kwan-Liu Ma in UC Davis Computer Science and the Center for Visualization to integrate the AI algorithm into an easy-to-understand platform that both clinicians and patients can access and make health care decisions accordingly.
The project had promising results, revealing that patient-reported data is surprisingly accurate for predicting readmission among all patients. The project has also demonstrated that patient-reported data can be very effective for identifying which patients would benefit most from telemonitoring. This is a valuable conclusion because telemonitoring is comparatively expensive to administer and this result helps health care providers to use this limited resource to maximum benefit.
The initial research results demonstrated that predictive learning algorithms developed in artificial intelligence research can be used to analyze this data and uncover early warning signs for individual patients that would otherwise be very difficult to discern. In addition to demonstrating a helpful tool for heart failure patients and their care providers, the preliminary research indicates that some measures that are considered subjective, such as the patient’s sense of wellness, can be surprisingly accurate indicators of the need for further treatment. The new knowledge generated by this insight may prove valuable in a wide range of health care situations.
This project is an inspiring example of a HIBAR project. The research required the integration of new techniques in artificial intelligence using large and complex data sets with the practical needs of a particular segment of the health care system, with a goal of achieving considerably better health outcomes. To achieve these dual goals, academic experts in health care, data science, and visualization partnered with clinicians, patients, and other stakeholders. As a result of their efforts to better predict readmission in an easily accessible manner, patients and clinicians can make appropriate treatment decisions long before hospitalization is required.